Fraud Detection in Data Mining: Strategies, Techniques, and Best Practices
Financial fraud is a major problem that costs institutions and consumers billions yearly. Account fraud, credit card fraud, insurance fraud, and scams are just a few of the many types of financial fraud that can occur.
Scammers can easily drain individual accounts or run up tens of thousands of dollars on credit cards. Worse yet, organized crime rings can execute elaborate schemes and steal millions of dollars.
Fraud detection is essential for minimizing risk for institutions. And, data mining plays a key role in improving fraud detection. Data mining uses machine learning and other techniques to uncover insights and patterns hidden deep in the terabytes of data your organization produces every day. Data mining enables institutions to better spot patterns of fraudulent behavior and prevent losses.
What is data mining?
Data mining is the process of finding patterns and relationships in large data sets. It can be used with data from a variety of sources, such as customer transactions, social media posts, or other financial data. Data mining can be used to identify trends, predict future behavior, and make better decisions.
With data mining, unifying data and extracting intelligence is now possible using modern technology. It allows businesses to uncover new insights with more granularity than ever before. This process makes it easier for companies to innovate, make smart business decisions, and create strategies that lead to long-term success.
What is fraud detection in data mining?
The right data mining solution can help institutions reduce the average expense of fraud detection by nearly 50%. By analyzing large volumes of data and identifying patterns, anomalies, or suspicious activities that may indicate fraudulent behavior, companies can save time and money—as well as protect their reputations.
Data mining helps prevent fraud in a few key ways, including the following:
1. Identifies patterns that indicate fraudulent behavior.
Data mining can be used to identify patterns of fraudulent behavior that may not be immediately obvious. For example, a data mining solution could identify suspicious patterns of credit card fraud by large purchases made in a short period of time or purchases made in different countries.
2. Predicts future fraud.
Data mining can look for trends in historical data to forecast future risks. For example, data mining could be used to predict which customers are most likely to default on their loans by looking at their past payment history and other factors.
3. Prioritizes fraud investigations
Data mining can help teams prioritize fraud investigations by identifying cases that are most likely to be fraudulent. For example, cases that involve large sums of money or cases that involve repeat offenders should be first priority for fraud detection teams.
Data mining is a powerful tool for fraud detection, but it has limitations. Companies should be aware of the best practices and challenges of using data mining to keep the risk of fraud at a minimum.
4 Types of fraud can be detected through data mining
Fraud detection in data mining can help reduce the risk of various financial crimes. With the right fraud-detection datasets and tools, institutions can make progress toward preventing these common types of fraud.
Credit card fraud
Credit card fraud detection is a system for uncovering fraudulent credit card transactions. Its goal is to provide the proper information to credit card companies so that true cardholders are not charged for transactions that they have never actually done. Data mining algorithms can be used to identify patterns of transactions in a credit card fraud dataset that are out of the ordinary, such as large purchases made in a short period of time or purchases made in different countries.
[Read more: 7 Steps For Preventing Credit Card Fraud]
Insurance fraud
Insurance fraud occurs when a claimant attempts to obtain some benefit or advantage they are not entitled to, or when an insurer knowingly denies some benefit that is due.
There are two types of insurance fraud: hard fraud, which occurs when someone deliberately fakes an accident, injury, theft, arson, or other loss to collect money illegally from insurance companies; or soft fraud, in which normally honest people often tell “little white lies” to their insurance company to file or maximize a claim.
Data mining can be used to identify patterns of insurance fraud by looking for claims that are out of the ordinary, such as claims that are made for injuries that are not consistent with the reported accident.
Bank fraud
Bank fraud encompasses various fraudulent activities: from wire fraud to credential stealing to account takeover fraud. Data mining can be used to identify patterns of bank fraud by looking for transactions that are out of the ordinary, such as large withdrawals made from accounts that do not typically have a lot of activity. It can also be used to reduce the risk of investment fraud, such as large purchases of stocks that are not well-known or that are not expected to perform well.
Healthcare fraud
Data mining algorithms can analyze medical records, billing data, and patient profiles to identify unusual patterns or outliers. With these datasets, data mining is able to identify patterns of healthcare fraud by looking for claims that are out of the ordinary. This can include claims that are made for services that were not actually provided or claims that are made for services that are not covered by insurance.
Common data mining techniques and tools for fraud detection
Fraud detection in data mining uses a few core techniques to uncover potential instances of fraud.
- Decision trees: A decision tree uses a type of supervised learning algorithm to classify data. Decision trees build models to identify patterns of fraudulent behavior.
- Neural networks: These machine learning algorithms can be used to learn complex patterns in data, as well as to build models that identify patterns that are not easily identified by other techniques.
- Association rule mining: This technique can find patterns of co-occurrence in data and build models that identify patterns of fraudulent behavior that are often associated with each other.
- Support vector machines (SVMs): Like decision trees, SVMs are a type of supervised learning algorithm that can be used to classify data. SVMs are particularly good at identifying patterns that are not linearly separable.
- Logistic regression: This type of supervised learning algorithm can predict the probability of an event occurring. It’s used to build models that predict the probability of a transaction being fraudulent.
These techniques are incredibly useful for detecting fraud, especially compared to manual methods. However, data mining is not a perfect solution. Online criminals are constantly evolving their techniques, and institutions must constantly revisit their data mining models to ensure they are up-to-date.
Additionally, data mining can only identify patterns of fraudulent behavior. You must have a human review of the results of data mining to ensure that any potential fraud cases are investigated thoroughly.
How can Fraud.net help?
The unified data platform at Fraud.net allows companies to connect their disparate, siloed data, append to our network data to give it context and run the datasets through machine and deep learning algorithms to give it actionable and automatable paths forward.
Our Collective Intelligence Network is the largest global anti-fraud consortium and platform-as-a-service for enterprises across industries, especially those vulnerable to financial fraud. Through collaboration, big data, and advanced AI, we enable our members to identify and stop fraud attempts long before they have to experience a loss.
The Fraud.net platform is designed for fast-growing digital leaders. Integrate quickly, test aggressively, and reap the rewards of continuous improvement.
Find out more about Fraud.net’s data mining tools and help your business lower the risk of fraud. Get in touch with us today for a free demo.
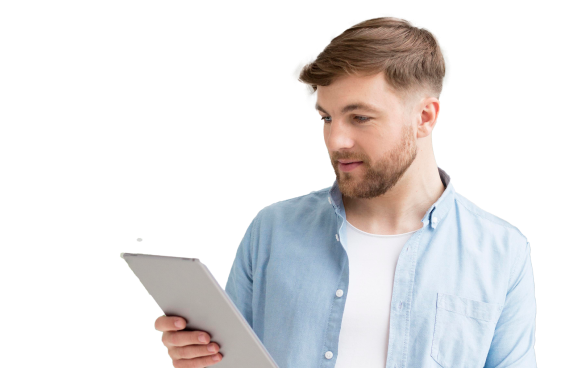
You might be interested in…
Get Started Today
Experience how FraudNet can help you reduce fraud, stay compliant, and protect your business and bottom line