How Technology Can Improve Anti-Money Laundering Systems
Banks can use advanced tools like AI, deep learning, and machine learning to analyze and predict money laundering schemes, and comply with KYC standards to avoid fraud.
Criminals rely on advanced money laundering schemes to store and access cash from illegal activities. When such a scheme is successful, criminals have access to money to fund more illegal activities. On a large scale, these plots have disastrous outcomes for the safety of the communities affected by crime. They can hurt the private sector, financial markets and the economy. Financial institutions are an important line of defense against these schemes. Their role is to spot dubious transactions. However, false positives can get in the way of an efficient anti-money laundering system.
The scope of money laundering
The UN estimates that money laundering accounts for $1.6 trillion each year. This amount corresponds to 2.7% of the global GDP.
Criminals use plots that are becoming more advanced. Plus, the volume of money laundered is going up. The Russian Laundromat is one example of how criminals are developing complex schemes to launder money.
The Laundromat operated between 2010 and 2014, scheming $20.8 billion out of Russia. Police suspect that 500 people were involved. Additionally, they found over 100 bank accounts linked to this network.
Schemes like these are hard to detect. Criminals typically place money into the financial system before creating layers to make it hard to trace. Their goal is to integrate the money into assets they can access.
Top challenges for anti-money laundering systems
Financial institutions have to monitor a growing volume of data. For instance, KYC AML, or Know Your Customer, guidelines require banks to verify the identity of their clients. This process is getting harder since clients have a more complex digital footprint.
Banks also need to monitor more products than ever before, including more digital solutions. Indeed, while the number of branches went down, banking activity is on the rise, with the number of deposits increasing by more than 200% in the past decade.
Criminals tend to favor wire transfers, which is challenging since utilization rates are increasing for these products. In fact, the Fedwire Funds Service saw a growth of more than 9% for the number of wire transfers in 2020.
Besides having more data and more products to work with, financial institutions are also facing more regulatory pressure. The Anti-Money Laundering Act of 2020 introduced some important measures for tackling this issue. Specifically, they include new reporting requirements and codifying the approach to compliance.
However, these measures also translate into a more complicated regulatory environment. Money laundering plots often involve banks in different countries. Banks have to keep up with global regulations too and must document compliance.
Lastly, fraud prevention teams often deal with high volumes of false positives. Reviewing false positives slows down AML programs. It also increases mistakes. Moreover, customers tend to be unhappy with the resulting delays in service.
How to improve anomaly detection for AML programs
Current AML systems often use customer data. They look for anything unusual, typically by analyzing the source of the funds. This approach has some limits though. It can miss complex schemes. Besides, criminals can reverse engineer it.
It’s hard to keep up with the amount of data available for each transaction. Anything that looks different can trigger a false positive. As a result, anti-money laundering teams are often overwhelmed. To overcome this, financial institutions require:
- Analysis. Richer insights and better tools to help find odd activity.
- Compliance. Automated use of KYC to make sure all transactions are filtered.
- Prediction. Monitoring of industries and areas with high risk.
Advanced tools like AI, deep learning and machine learning are perfect for banks for these reasons.
These tools can improve compliance with AML regulations because they can sift through larger volumes of data. AI achieves better results by leveraging more data points. The goal is to get a full picture of the transaction. This approach greatly reduces false positives.
With AI, anti-money laundering teams can access risk scores for each transaction. It's an intuitive tool. Employees can spot high-risk transactions easily.
Most importantly, they can focus on the most suspicious transactions while the system handles the rest.
How Fraud.net can help
Money laundering is a growing threat. Criminals are using more complex plots. So, financial institutions need to look for new solutions.
Fraud.net has a portfolio of fraud prevention solutions for financial institutions. Here’s how we can help with your AML system:
- AI. We offer AI solutions for your unique needs. The benefits include fast implementation, cost-effective fraud prevention and a notable reduction in false positives.
- Risk scoring. Fraud prevention experts can see a risk score for each transaction. It’s a valuable data point for prioritizing reviews and making decisions.
- Consortium data. Build a better KYC AML by using our consortium data. Even if a client is new to your system, you’ll get valuable insights based on previous interactions with other financial institutions.
Find out more about how Fraud.net can help you build a better anti-money laundering program. Schedule a demo today to see our AI solutions in action.
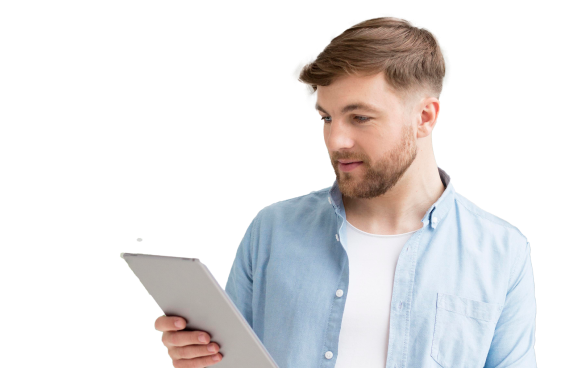
You might be interested in…
Get Started Today
Experience how FraudNet can help you reduce fraud, stay compliant, and protect your business and bottom line