How the World's Largest Banks Use Advanced Graph Analytics to Fight Fraud
Advanced graph analytics provides deeper insights, complementing BI, and helps organizations preempt and prevent potential fraud while protecting customers.
Excerpted from an RTInsightsArticle
Financial services organizations are both powerful and vulnerable. As a result, they are often a target for fraudsters and cybercriminals. Merchants and financial services organizations will spend $9.3 billion annually on fraud detection and prevention by 2022. Global online payment fraud (also called CNP or “Card Not Present” fraud) alone will cost merchants $130 billion in just five years (from 2018 to 2023). The latest report from LexisNexis also indicates that fraud attempts have increased significantly among financial services firms during the past year, with more than twice the number of attempts and an 85 percent increase in fraud success rates.
As the scale and scope of fraud has increased to encompass digital and mobile customer platforms and devices, financial services organizations are placing “fraud prevention and detection” at the top of their priority lists. In fact, five of the ten largest global banks and two of the world’s largest payment card companies have turned to advanced analytics in graph for their anti-fraud initiatives. Gartner analysts have highlighted Graph Database & Analytics as a “top 10 trend for data and analytics,” with an estimated annual growth of 100 percent annually through 2022. This technology helps analyze, detect and visualize complex data patterns – patterns that indicate the potential for fraud.
Basically, graph analytics is a set of analytic techniques that allow you to “drill down” into complex interrelationships among organizations, people and transactions. For example, a leading U.S. multinational investment bank is using advanced graph analytics to improve its fraud avoidance initiatives, specifically fraud detection for debit and credit cards. The organization is adding graph analytics to its machine learning system to find data connections between “known fraud” credit card applications and new applications. As a result, the bank can identify more questionable patterns, expose fraud rings and shut down fraudulent cards faster. The bank will then save millions of dollars annually. Also, the world’s largest payment card provider chose advanced graph analytics for payment fraud detection and merchant credit management. The company, which had been using RDBMS (relational database management system), needed technology that offered deep pattern analytics and real-time update capabilities for its anti-fraud work.
Fraud detection requires understanding connections and identifying anomalies in links among people, transactions, payment methods, locations, devices, times and more — and working with huge and ever-changing datasets to do this in real time. The world’s most innovative financial services organizations are opting for advanced analytics in graph, or advanced analytics in connected datasets (“graph” refers to connected data). Advanced analytics in graph allows a system to process a payment while understanding how a transaction is connected to different datasets. Then, a “next best action” is offered up; in the case of fraud, this may be “reject payment” while marking a user with a “zero” trust score. But there is a significant difference between regular analytics and advanced analytics – especially when it comes to detecting fraud.
Learn More
- Read the Full Article
- Visit the Banking page on our website
- Speak with a Fraud Prevention Specialist
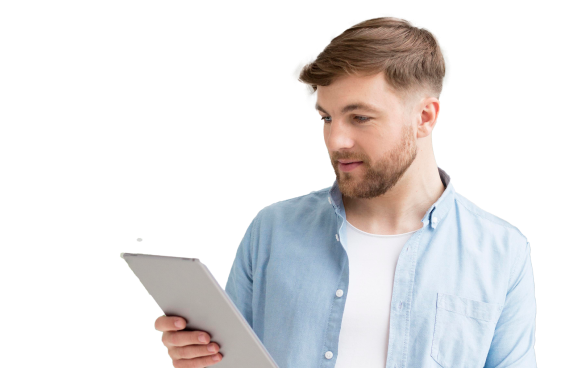
You might be interested in…
Get Started Today
Experience how FraudNet can help you reduce fraud, stay compliant, and protect your business and bottom line