Podcast: PreCrime Fraud Detection: How AI Is Being Used to Identify Criminals Before A Crime Is Committed
Stopping fraud before it is committed may seem far-fetched, but AI and other technological advances are quickly moving us closer.
[Auto-generated transcript]
Tom: Well, let's not kid ourselves. We are arresting individuals who have broken no law.
Colin: But they will. The commission of the crime itself is absolute metaphysics. The pre-cogs see the future and they're never wrong.
Tom: But it's not the future if you stop them.
Colin: Isn't that a fundamental paradox?
Tom: Yes, it is. You're talking about predetermination, which happens all the time. [noise] Why did you catch that?
Colin: Because it was going to fall.
Tom: You're certain?
Colin: Yeah.
Tom: But it didn't fall. You caught it.
David: That clip, featuring Tom Cruise and Colin Farrell, was from the prescient 2002 movie, Minority Report, discussing the nature of causality and prediction. This conversation was specifically in the context of the crime of murder, predicting it and stopping it, which was allegorically represented by a red ball that Cruise was rolling along a curved desktop for Farrell's character to catch. Minority Report showed the potential ramifications of precrime capability, from the positive, a steep reduction in crime, to the negative, false positives. If you haven't watched it yet, it's a great movie and it's aged really well, I highly recommend it.
Anyway, here in 2020, stopping crime before it is committed has arrived, for fraud at least, well ahead of 2057, which is when the story of Minority Report was set. Unlike the movie, which leverages the skills of three psychics in a Jacuzzi -- it's complicated -- companies like Fraud Net are leveraging predictive analytics and sophisticated behavioral algorithms to identify potential fraudsters before the fraudulent transactions occur.
Today we'll be speaking with a man who is very arguably the Tom Cruise of the fraud prevention industry, Michael Fossel. Michael is the head of client success at fraud.net, and we'll be talking about how technology spots precrime and fraud, and the ethical considerations.
Thanks for joining us today, Michael. Tell us about the new technology that you're working on that is looking at device information as a way of flagging fraudsters before a fraudulent transaction occurs.
Michael: We're able to gain deeper insights into the actual device -- whether that device was using a proxy or a TOR, or if it was coming from a country that's highly associated with fraud.
And why this is really important is that the closest, most distinct fingerprints that you can have to link to an identity, more so than an address, phone number, or email address, as I'm sure you're well aware -- you know you are your phone.
We track the device as different events are occurring with that device. That could be a login attempt. It could be a sign up, whether you're signing up for a new service, signing up for a new credit card, or even doing a financial transaction or changing your account information. We're now able to track the device used when all of those events, or any of those events are occurring.
David: So, how is the technology identifying fraudsters before they commit fraud?
Michael: There's a few indicators, that are just common sense things, like -- is this coming from a country or city, or something like that, that's never been associated with that individual user?
Typically, I myself, use my device in New Jersey and sometimes New York or I could be traveling. But if all of a sudden that device is now registering from Moscow, Russia, that could be an indication that something has gone awry.
Furthermore, you know, we're also looking at behavioral aspects, like -- am I my purposely trying to mask where I am? Am I using a proxy or TOR node? Or do I have the behavior of a bot? Meaning that it's taking two milliseconds for me to log in, when typically that's a much longer process.
All of these things kind of put together can be anomalous in the sense that this is unlike the previous behavior that the customer has logged with any of our clients.
So, when we take that information, it obviously will score higher as being of risk because it's anomalous to their normal behavior. From there, we look at other trends as they're actually getting closer to completing a financial event.
David: These operations take place in a fraction of a second. What's the advantage to flagging fraudsters prior to the purchase versus just at the moment of purchase? Or is what's taking place really happening in a fraction of the second before the purchase?
Michael: Yeah, I mean, it is happening that quickly. I think a good analogy would be the idea of someone knocking on your door, and not letting them in. Versus someone knocking on your door, you letting them in, and then five minutes later, they rob you. So the quicker you can identify that -- “that knocks sounds different”, or “I don't like something about how they're requesting access”, or “there's some anomalous behavior” -- if you can detect that sooner, you put your company at less risk, and the good customer at less risk.
Obviously, there's ways to verify the person behind the door if it really is them, such as adding a Captcha, or putting some form of authentication in place.
But really, that is why precrime, and detecting these things the second you're aware of it, instead of waiting till the last minute when you're more susceptible to let something go through.
David: What kind of a return on investment does this provide over technologies that are Just flagging the fraudsters right at the moment of purchase?
Michael: I'd say is some of it is invaluable in the sense that it's creating better customer experiences for good customers, and weeding out fraudsters at an earlier stage.
So I think that that piece allows for less bad transactions to even be evaluated. One of the big things within a fraud department is how many transactions or events they're really looking at per day. How much time spent on investigating or verifying good transactions versus bad transactions.
Now, if we can prevent a user or some anomalous behavior in this, using the same sense of, you know, someone coming from Russia that's using a TOR node, or all these things that -- we then don't have to take a second look at that. We don't need to spend the man hours to investigate that further. That's something that -- 10 minutes before the transaction occurs, if we knew that, we wouldn't accept that. We wouldn't even let that go through.
So, it helps in that situation for sure. It controls who's getting access to these certain things. And even from the beginning, when we're talking about using device shields for deciding who you even do business with, it's much better to know that someone who's attempting to get a credit card from you or taking credit out, a use case, but even just working at signing up to be in your e-commerce company or anything like that, if we've seen bad behavior from them across our network and their associated with fraud with other users, or they're logging in in some way that is just indicative of 99% of the time when we see this it turns out to be a fraudulent behavior later, if we can prevent people like that from even attempting to do business with you, that affects your bottom line tremendously.
David: Right. You you anticipated my next question. In cases where you find a fraudster who is misusing a legitimate customers identity -- I mean, obviously you want to find that out as early as possible, whether they have made a purchase or not -- but the group I was curious about is the people who, as soon as they register, you're like -- something's off here -- new customer, who...
So, this isn't just detecting cases of say, identity theft or credit card number theft, for example. This is also detecting, like when people are creating outright fraudulent accounts right from the get go, right?
Michael: Of course, of course. Fraudsters are smart, innovative people. And if they're not let in the front door, they're going to try to get in the side door and the back door and through the window and all these things. But at the end of the day, they're using similar behaviors to get access to each entry point.
We're looking for that. And stopping it before they are even allowed to transact with you is obviously better, of course.
From an account takeover perspective of someone getting my password or my credentials and logging in from a different device in a different area, things like that -- that's what we can stop, and that's what everyone is trying to stop.
But if we take a look at plugging the leakage a little earlier, we're getting into accounts and log in attempts, that just lessens the likelihood of -- not to pick on Russia here, but, things like that or behaviors like that happening.
David: We're talking about precrime, and The Minority Report, the issue in that movie was the idea that you may have a great deal of certainty that someone's about to commit a crime, but in fact, in rare instances, they may not.
It doesn't sound like there's the same ethical concern here, because you either have somebody who's stealing someone else's identity, which is a crime as soon as that occurs whether or not they decide to commit fraud with it.
But what happens in the case -- are there cases where a legitimate customer signs up, they trip the switch, and they're labeled as a fraudster? What's the consequences for them? How is it resolved?
Michael: We have mechanisms in place where, if something looks off, nine times out of ten, this is something that turns out to be fraudulent. But on that one chance, maybe it's not. We have tools in place where, either through automation, or through a manual investigation, we have the ability to authenticate the user at an account level. So whether that's sending a text message or SMS or an email or reaching out to who the customer is, to verify, hey, was this you? And there's a number of ways we can do that, which really reduces the friction points.
It's been a while since I saw Minority Report, but I don't think they say, hey, were you thinking of doing this? Yes or no?
So there is that aspect to do no harm. Because we really don't want a company to lose out on revenue, for us to reject a good, honest paying customer from buying what they want to buy or using their card where they want to use it. But at the same time, not creating friction in that situation while limiting the overall risk of the company.
So there is a balance there. But I think from the standpoint of doing no harm, we do -- I don't want to say "tread lightly" -- but we do have checks and balances in place to confirm things before taking a final action, if our customers want to act on it.
David: Thank you, Michael. My name is David Zweifler, and I've been speaking with Michael Fossel, director of client success at Fraud.net about how AI and Predictive Analytics are being leveraged to identify fraudsters, precrime in an e-commerce setting.
This podcast is an excerpt of a webinar conducted with Michael, where he goes into greater depth on the technology called the Vice Shield and how it works.Fraud.net has also recently published a highly informative e-book on AI and precrime fraud detection.
You can access both through links in the description.
You've been listening to the podcast for fraud.net. Fraud.net uses collective intelligence and machine learning to make all digital transactions safe.
Learn more at fraud.net.
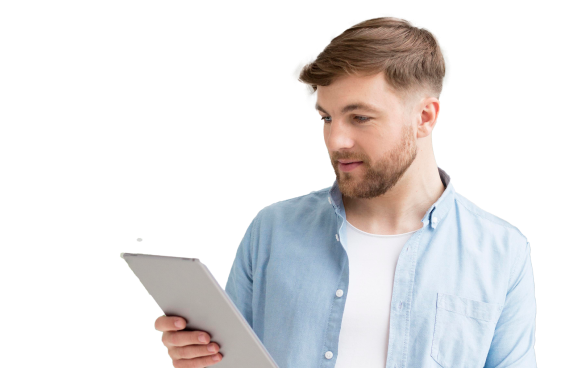
You might be interested in…
Get Started Today
Experience how FraudNet can help you reduce fraud, stay compliant, and protect your business and bottom line