Using Machine Learning To Prevent Identity Theft
Leverage AI and machine learning for fraud detection to combat rising identity theft.
VentureBeat reports that the rise in identity fraud set new records in 2022. “Last year alone, businesses lost $20 billion to synthetic identity fraud, $697 billion from bots and invalid traffic, and more than $8 billion from international revenue share fraud (IRSF),” reported the tech outlet.
The costs of identity fraud are becoming untenable for businesses and financial institutions. Tackling identity fraud requires better solutions that employ machine learning: computer algorithms and statistical models that perform predictions and specific tasks using inferences and patterns.
Machine learning for fraud detection allows algorithms to make immediate decisions on new transaction decisions. Over time, these algorithms ‘learn’ from the outcomes of the data set and can self-correct to make increasingly accurate predictions going forward.
Identity theft and other fraud attempts are often successful because fraud prevention analysts are overwhelmed by the proliferation of threats. Machine learning can alleviate some of the manual effort required to identify identity fraud, improving efforts to prevent these threats from coming to fruition.
In this article, we’ll look at how machine learning for fraud detection improves the identification and response to potential instances of identity fraud. We’ll also discuss how these solutions can be deployed in your organization.
5 Common types of identity theft
At Fraud.net, we define identity theft as “the act of accessing and acquiring elements of another person’s identity (i.e. name, date of birth, billing address, etc.) in order to commit identity fraud.” This definition can cover a broad range of activities. As a result, it’s helpful to zero in on five instances of identity theft that are most commonly faced by businesses and banks.
1. Financial identity theft
In financial identity theft, a criminal uses someone else’s identity for financial benefit. This can include activities such as accessing someone’s bank account, using their credit card, opening a new line of credit and making purchases by impersonating the individual victim. Financial identity theft is increasingly common with the rise of e-commerce and online banking.
2. Synthetic identity theft
This tactic is one of the fastest-growing types of financial crimes and one of the most effective methods of identity theft. It combines real identity information — such as someone’s name and address — with fake information. In this method, fraudsters use real birthdates and other information to create fake profiles, which can be used to apply for loans, credit cards, and other financial crimes.
3. Medical identity theft
Medical identity theft is less of a concern for banks and nonmedical businesses, but it’s still very prevalent, particularly in the wake of the pandemic. These schemes involve using the victim’s information in order to access health care services. A criminal will steal an individual’s name, birthdate, SSN, and other details in order to get prescriptions, access medical services, or get medical supplies such as hearing aids or wheelchairs.
4. Account takeover identity theft
When someone gains access and takes control of one or more accounts without the user’s knowledge or permission, they’ve perpetrated account takeover identity theft. When they gain access, they can use the victim’s account to make fraudulent transactions and transfer money.
5. Criminal identity theft
Finally, criminal identity theft occurs when an arrested person gives someone else’s name instead of theirs. Like medical identity theft, most institutions and banks don’t need to be concerned with preventing this type of identity theft. These are just a few of the most common forms identity theft can take; there are dozens of other iterations and creative ways that fraudsters exploit their victims’ personal information. Because the form this crime takes is ever-evolving, it makes it extremely difficult for fraud prevention analysts to consistently and accurately spot identity theft as it’s happening.
What are the most common signs of identity theft?
Analysts tend to use a few heuristics, or shortcuts, to spot identity theft as quickly and accurately as possible. Nevertheless, traditional methods for finding the common signs of identity theft tend to fall short. By one estimate, existing fraud discovery models are ineffective at detecting between 85% to 95% of likely synthetic identities.
To prevent identity theft, businesses need to be able to answer two questions:
- Is the customer real?
- Is the customer who they claim to be?
Many businesses try to answer these questions by tracking transactions and giving each one a fraud score. Unexplained charges or chargebacks, uncharacteristically missed payments, or payments from new or unidentified devices could all be common signs of fraud. By identifying traits and historical trends associated with suspicious behavior and fraudulent orders, fraud prevention tools can try to detect fraud in their transactions to avoid major profit losses.
Some tools focus on the second question, using identification verification as the primary form of prevention. “ID scanning solutions have various strengths; some simply scan an ID’s barcode, whereas more robust software performs forensic and biometric tests to ensure that an ID is not forged,” wrote Tech Beacon.
While these signs provide a good baseline for spotting identity theft, machine learning tools provide a new, more powerful way to prevent identity theft at scale.
How to use machine learning for fraud detection
Machine learning shortens the time it takes to spot a threat and improves the results of identity theft protection.
“The goal is to better train supervised machine learning algorithms to identify anomalies not visible with existing fraud detection techniques while supplementing them with unsupervised machine learning exploring data for new patterns,” wrote VentureBeat. “Combining supervised and unsupervised machine learning in the same AI platform differentiates the most advanced vendors in this market.”
Transaction AI and Application AI, two machine learning tools from Fraud.net, show how machine learning can help improve fraud prevention at scale. These tools operate in four key phases:
- Collect user, event, and device information for each transaction;
- Enrich this data with collective intelligence, customer ML models, and the app store;
- Assess with case management, analytics and reporting, and real-time analysis;
- Decide whether a transaction poses a risk of identity fraud.
Transaction AI has led to four times more accurate fraud detection and a 53% reduction in fraud case investigations. Like other machine learning tools, the goal is to reduce the rate of false positive cases, prioritize the right cases for the fraud prevention team to resolve, and provide a seamless experience for the customer. And with Fraud.net’s layered approach, companies can build an AI-powered risk intelligence solution designed to handle each stage of the transaction lifecycle.
Interested in using machine learning for fraud detection?
Schedule a demo with one of our solutions consultants today to learn how you can upgrade your fraud prevention.
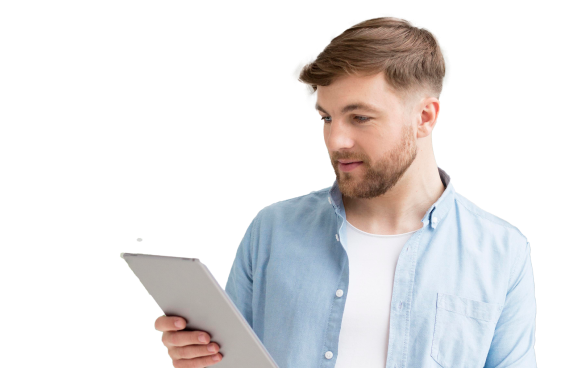
You might be interested in…
Get Started Today
Experience how FraudNet can help you reduce fraud, stay compliant, and protect your business and bottom line